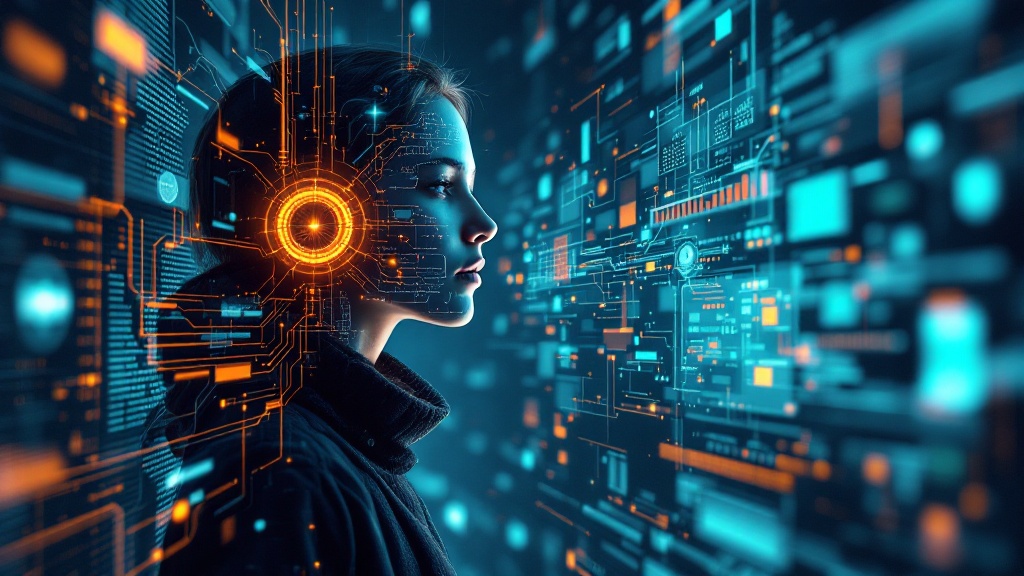
Voice AI and Sentiment Analysis for Sales: Insights into Gaining the Competitive Edge
Voice AI has rapidly evolved from a novelty to a strategic asset. For sales enablement use cases in particular, understanding tone, context, and customer sentiment can be the difference between closing a deal and losing a lead. Tools powered by Voice AI with sentiment analysis are helping organizations bridge this gap by enabling deeper customer insights, personalized experiences, and faster decision-making. As we look to the future, two elements emerge as critical: a high quantity of quality of voice data, and using it to train LLMs
The Rising Importance of Voice AI in Sales
Voice AI is transforming how companies engage their customers. It is now common to find sales representatives using Voice AI solutions that transcribe calls in real time, highlight potential customer pain points, and suggest strategic responses to objections. Here are a couple of compelling reasons why it’s catching on:
- Driving Customer Engagement: By leveraging Voice AI and LLMs, companies can interact with prospects more efficiently; whether through virtual assistants or real-time conversation coaching. This immediate feedback loop helps sales professionals refine their pitches and maintain customer interest.
- USE CASE: A sales representative uses a voice assistant to monitor customer sentiment during a demo call. When the tool detects hesitation, it suggests offering a discount or focusing on a specific feature to address the customer’s concerns.
- Streamlining the Sales Process: Automated call analysis can free up hours of labor each week by summarizing meetings, surfacing key insights, and updating CRM records. This can cut down repetitive administrative tasks so sales teams can focus on building relationships and closing deals.
- USE CASE: TwinsAI integrates with a customer’s CRM to automatically transcribe calls, extract key action items, and automatically update the CRM with next steps. Each interaction improves future calls as the AI refines its recommendations, ensuring that sales teams stay on track without manual input.
Role of Sentiment Analysis in Voice AI
Sentiment analysis, particularly in voice, goes well beyond transcription and keyword detection. It interprets tone, pitch, and pace; all crucial elements that reveal a customer’s emotional state.
- Understanding Emotional Cues: A customer’s voice pitch or speech rhythm might indicate interest or frustration. With advanced AI sentiment analysis, organizations can capture and detect these nuances, helping salespeople recognize potential churn signals or upsell opportunities.
- USE CASE: During a product inquiry, the AI identifies frustration in a customer’s tone and alerts the representative to slow down and provide additional details about the product warranty.
- Personalizing Customer Interactions: When you understand if a customer is “annoyed” or “enthused,” you can tailor your message accordingly. For instance, if a prospect sounds hesitant, reps can switch to a more consultative tone, or if they detect excitement, they can move straight to discussing pricing and next steps.
- USE CASE: Real-time analysis suggests alternative phrasing when it detects customer skepticism, helping the rep steer the conversation positively.
Fragmentation of Voice Data: Challenges and Opportunities
While Voice AI offers numerous benefits, the industry faces a significant challenge: fragmentation. Organizations often store customer data in disparate systems, such as call center software, CRM platforms, cloud storage, individual reps’ call recordings, and more.
- Why Voice Data Is Scattered: Each department may manage its own recording solutions, or each region might rely on different vendors. This lack of a unified voice data management strategy leads to lost opportunities, as valuable insights remain trapped in different systems.
- Competitive Advantages of Data Consolidation: Organizations that successfully consolidate, label, and analyze their voice data enjoy a unique advantage. They gain a holistic view of customer interactions and can feed comprehensive datasets into advanced LLMs, improving accuracy, relevance, and predictive power. In an environment where proprietary data is gold, having a large corpus of well-structured voice data can significantly improve the quality in sentiment analysis and other AI-driven solutions.
At TwinsAI, our platform empowers customers by aggregating call data across departments, providing a unified dataset to train LLMs on your own personalized model. This ensures that TwinsAI evolves to meet unique customer demands and delivers actionable insights from day one.
Future Outlook: Voice AI and Sentiment Analysis
Looking ahead, the momentum for voice AI is expected to grow, driven by advancements in deep learning, more advanced language models, and improved speech recognition technologies.
- Emerging Technologies and Innovations: Expect more robust real-time analytics tools offering on-the-fly coaching based on content and sentiment. These tools may even simulate entire sales dialogues, predicting a customer’s reaction to different approaches. We also anticipate augmented or mixed-reality environments where voice AI assists in live negotiations or complex product demonstrations.
At TwinsAI, we are working on predictive call features where the platform anticipates a customer’s likely objections based on historical data and suggests counterarguments in real-time. - Ethical and Regulatory Considerations: As voice data handling becomes more comprehensive, privacy, data ownership, and compliance issues will intensify. C-level leaders should anticipate regulatory scrutiny and be prepared to implement frameworks that ensure ethical data usage while still driving business outcomes.
Strategic Recommendations for Leaders
- Build a Comprehensive Data Strategy: Formulate a plan to centralize voice data in a secure, privacy-compliant environment. This ensures you can analyze it holistically, rather than chasing fragmented insights across multiple platforms.
- Collaboration and Partnerships: Partner with specialized tech firms, cloud providers, or AI vendors to accelerate your data consolidation efforts. Collaboration can mitigate risks, reduce in-house development costs, and expedite time-to-value.
- Align Voice AI with Organizational Goals: Voice AI and sentiment analysis initiatives should tie back to broader strategic objectives, like boosting customer satisfaction, shortening sales cycles, or improving sales forecasting. This alignment helps justify the investment, facilitates cross departmental buy-in, and clarifies ROI metrics.
Voice AI and sentiment analysis are reshaping the sales landscape, presenting forward-thinking organizations with opportunities to drive productivity, improve customer experience, and make data-driven strategic decisions. However, these technologies require more than off-the-shelf deployments; they demand robust, high-quality voice data as fuel.
Fragmentation remains a major challenge, but by consolidating and curating data effectively, companies can gain a significant edge. Armed with comprehensive voice datasets, organizations can train advanced LLMs and build predictive models that elevate sales performance. For C-level executives, the time to act is now. By adopting a unified approach to voice data and aligning AI initiatives with core business objectives, leaders can ensure their organizations remain competitive in an increasingly voice-first world.